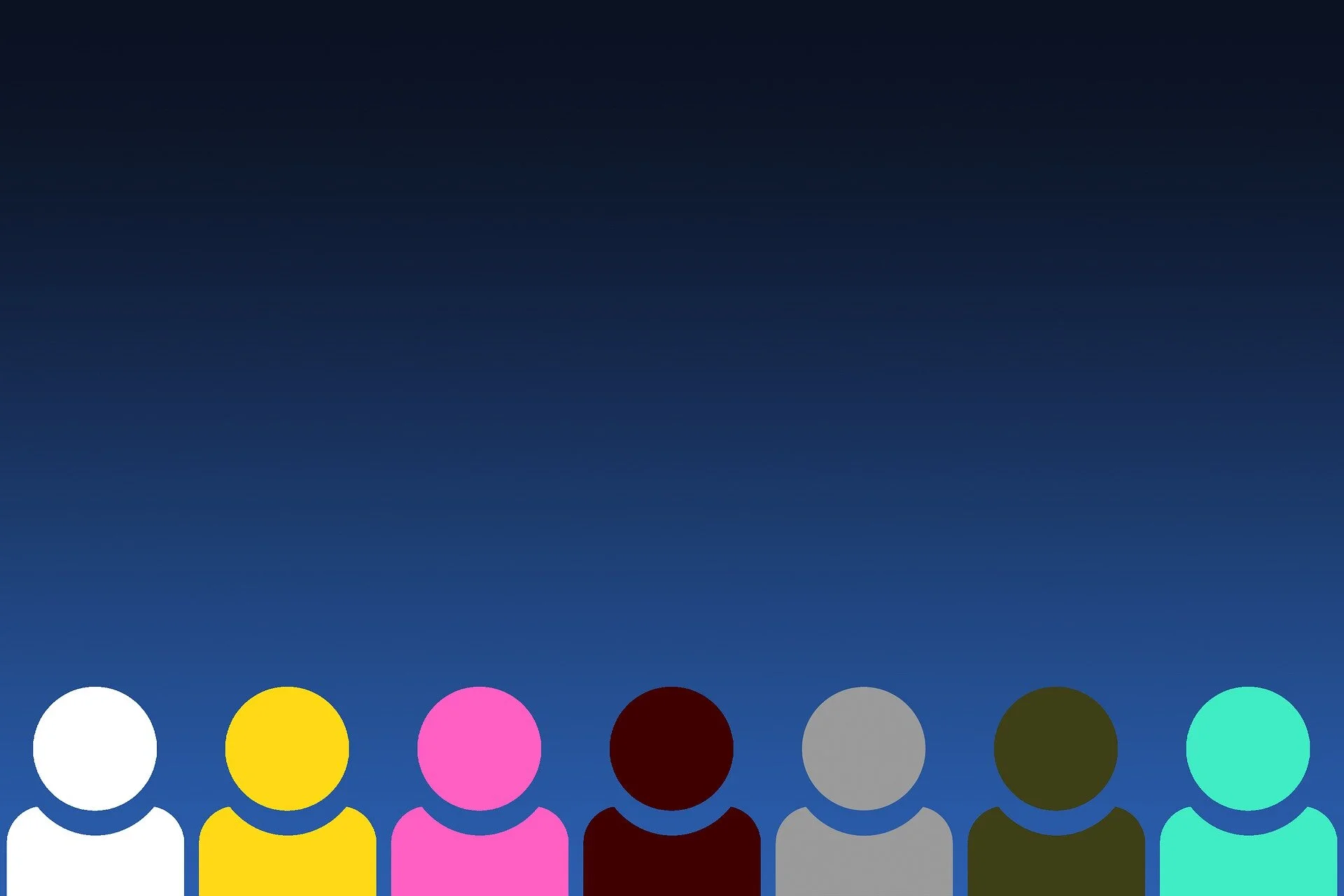
AI/ML bias identification and mitigation in medical image analysis.
An overview for medical imaging AI/ML researchers and others.
Brought to you by the MIDRC bias and diversity working group.
Last updated August 31, 2023
Credit: MIDRC bias and diversity working group
Selected literature
-
Citation: Drukker, Karen, Weijie Chen, Judy Gichoya, Nicholas Gruszauskas, Jayashree Kalpathy-Cramer, Sanmi Koyejo, Kyle Myers et al. "Toward fairness in artificial intelligence for medical image analysis: identification and mitigation of potential biases in the roadmap from data collection to model deployment." Journal of Medical Imaging 10, no. 6 (2023): 061104-061104.
-
citation: Banerjee, Imon, Kamanasish Bhattacharjee, John L. Burns, Hari Trivedi, Saptarshi Purkayastha, Laleh Seyyed-Kalantari, Bhavik N. Patel, Rakesh Shiradkar, and Judy Gichoya. "‘Shortcuts’ causing bias in radiology artificial intelligence: causes, evaluation and mitigation." Journal of the American College of Radiology (2023).
-
Citation: Zong, Yongshuo, Yongxin Yang, and Timothy Hospedales. "MEDFAIR: Benchmarking fairness for medical imaging." arXiv preprint arXiv:2210.01725 (2022).
-
Citation: Brown, Alexander, Nenad Tomasev, Jan Freyberg, Yuan Liu, Alan Karthikesalingam, and Jessica Schrouff. "Detecting shortcut learning for fair medical AI using shortcut testing." Nature Communications 14, no. 1 (2023): 4314.
-
-
Citation: Guldogan, Ozgur, Yuchen Zeng, Jy-yong Sohn, Ramtin Pedarsani, and Kangwook Lee. "Equal improvability: A new fairness notion considering the long-term impact." arXiv preprint arXiv:2210.06732 (2022).
-
Citation: Xiao, Yuxin, Shulammite Lim, Tom Joseph Pollard, and Marzyeh Ghassemi. "In the Name of Fairness: Assessing the Bias in Clinical Record De-identification." In Proceedings of the 2023 ACM Conference on Fairness, Accountability, and Transparency, pp. 123-137. 2023.
-
Citation: DeGrave, Alex J., Joseph D. Janizek, and Su-In Lee. "AI for radiographic COVID-19 detection selects shortcuts over signal." Nature Machine Intelligence 3, no. 7 (2021): 610-619.
Selected code
-
NIH’s NCATS challenged participants to create a solution that detects bias in AI/ML models used in clinical decisions. Note that the provided solutions were not necessarily directly related to medical imaging.
url: https://www.expeditionhacks.com/nih-bias-detection-gallery
-
Check back soon.
MIDRC-developed code
-
Check back later.
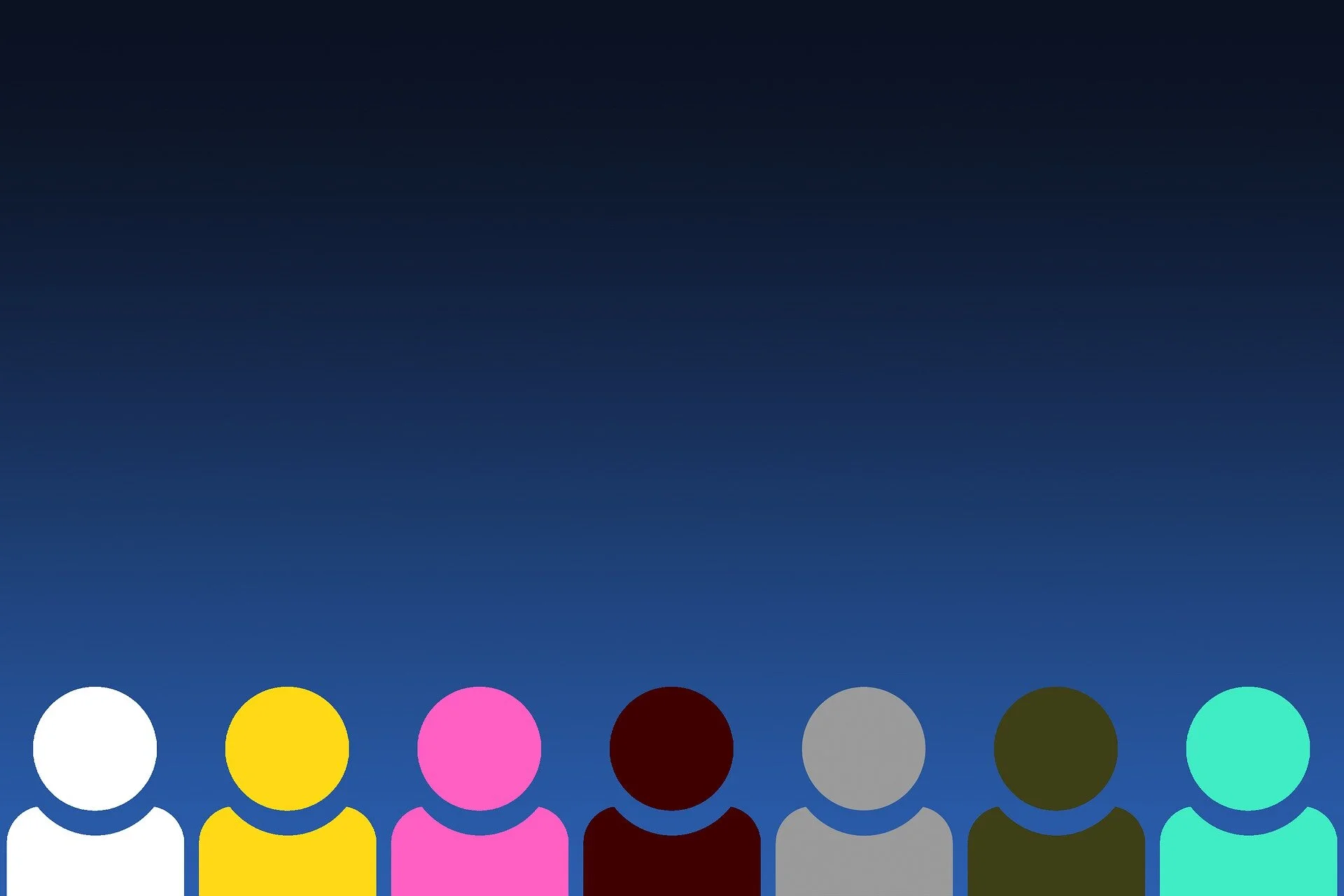