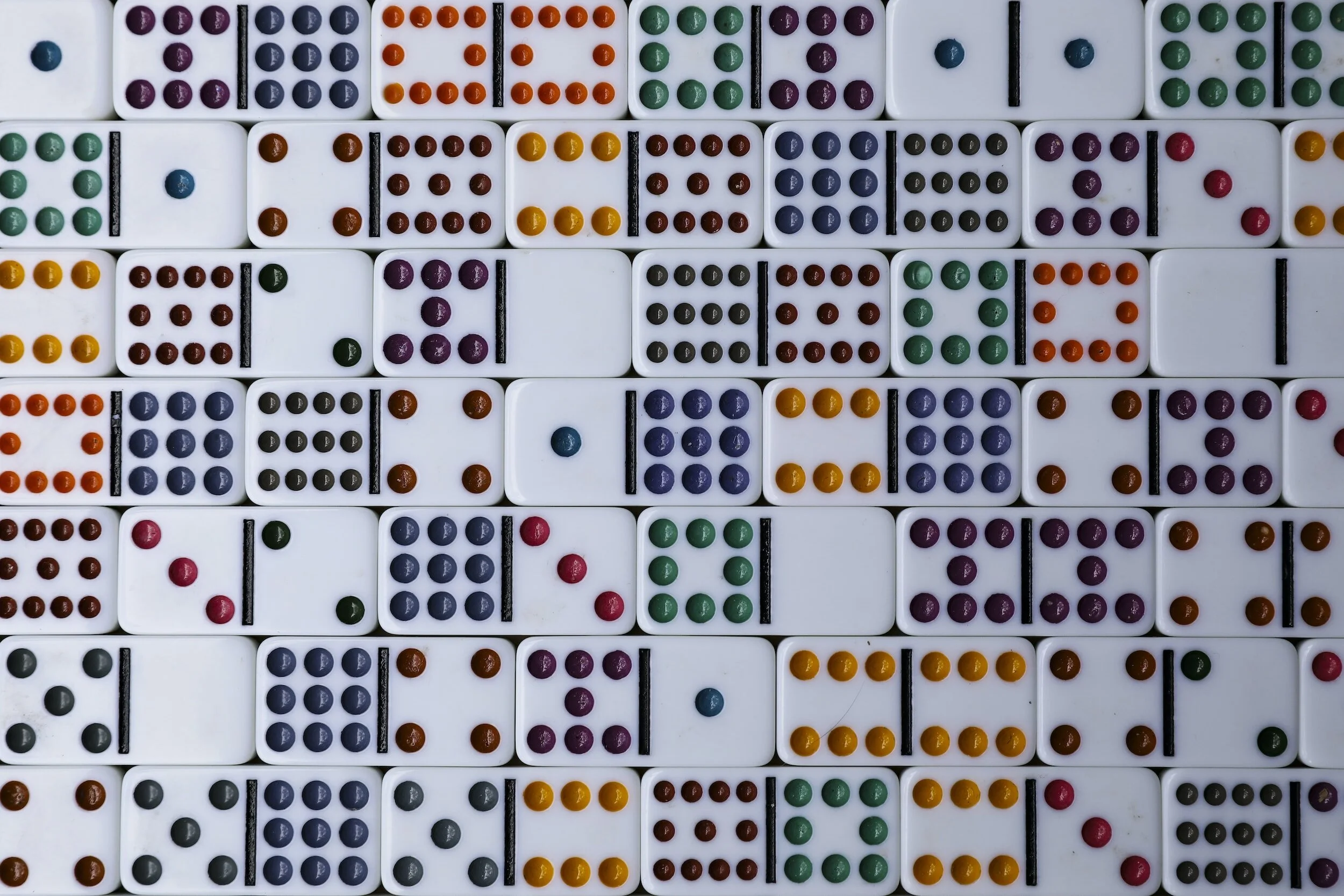
Tools, algorithms, methods, code.
Last updated November, 2024
Algorithms, methods, and code are continuously being developed within MIDRC. Please see below for a current list.
Check back often for updates!
See our ‘Web-based tools’ page for ‘plug-and-play’ tools for cohort building, indexing, performance metric selection, bias awareness, and more.
Featured:
The MIDRC diversity calculator is an open-source tool designed to assess and quantify the diversity within medical imaging datasets. By analyzing demographic attributes, this calculator helps researchers ensure that their datasets are representative of diverse populations, aiding in the development of more equitable and inclusive AI models in healthcare. The tool is hosted on GitHub, where users can access the code, contribute, and collaborate to improve its functionality.
Learn more about the diversity calculator in this short demo video, a MIDRC seminar recording, or the peer-reviewed publication.
The MIDRC DICOM harmonization mapping tool is an open-source utility aimed at standardizing and harmonizing DICOM files across diverse medical imaging datasets. By aligning and normalizing metadata and image attributes, including unstructured character string fields, this mapping table facilitates cohort selection in the MIDRC data commons and ensures the integration and interoperability of imaging data, providing consistency quality in large-scale research initiatives. It is available on GitHub for users to access and input suggestions for additional LOINC terminology.
Learn more in this MIDRC seminar recording.
RadGraph is a tool designed to support the development of AI models that understand and evaluate radiology reports. Combining a Python-based labeling tool with an annotated dataset, RadGraph enables researchers to extract medical terms and their relationships from unstructured text. It serves as a foundation for training and refining natural language processing (NLP) models specifically tailored to healthcare applications. RadGraph is hosted on PhysioNet, accessing the tool requires user credentials.
Explore RadGraph’s code and additional resources on its GitHub page. Pre-trained models and details about RadGraph are available on HuggingFace. RadGraph is described in several peer-reviewed publications, which provide deeper insights into its development and applications: ACL 2024 Findings: Overview of RadGraph’s advancements in radiology NLP. and EMNLP 2022 Findings: Key use cases and evaluation of RadGraph’s dataset.
The Stanford de-identifier base is a pre-trained model developed by Stanford's AIMI Center to automatically remove or obscure personally identifiable information (PII) from medical text. Hosted on Hugging Face, this tool is designed to ensure patient privacy by de-identifying sensitive data in clinical reports, enabling researchers to safely use and share medical documents for research and AI development without compromising confidentiality. The model can be easily integrated into workflows to enhance data privacy practices in healthcare.
Read more about the de-identifier in this peer-reviewed publication.
The RSNA DICOM anonymizer is a free open-source tool for curating, de-identifying and transferring imaging datasets. The Anonymizer program has versions for major OS platforms (MacOS, Windows, Linux Ubuntu) designed to perform "on-prem" de-identification of imaging datasets for use in research. Written in Python, using widely adopted libraries for processing medical images, Anonymizer is designed to be extended for specific project needs.
The generalized stratified sampling tool on GitHub is a resource for researchers looking to implement advanced sampling techniques in medical imaging studies. This tool offers a framework for stratified sampling, which helps ensure that samples are representative of various subgroups within a dataset. It supports the development of more robust and generalizable models by improving the distribution and diversity of sampled data, making it easier to analyze and interpret complex imaging datasets effectively.
Read more about the de-identifier in this peer-reviewed publication.
Complete list:
CRP=Collaborative Reseach Project, TDP=Tecnology Development Project, BDWG=Bias and Diversity Working Group)
-
The MIDRC Diversity Calculator is a tool designed to compare the representativeness of biomedical data. By leveraging the Jensen-Shannon distance (JSD) measure, this tool provides insights into the demographic representativeness of datasets within the biomedical field. It also supports monitoring the representativeness of datasets over time by assessing the representativeness of historical data. Developed and utilized by MIDRC, this tool assesses the representativeness of data within the open data commons to the US population. Additionally, it can be generalized by users for other diversity representativeness needs, such as assessing the similarity of demographic distributions across multiple attributes in different biomedical datasets.
Available at https://github.com/MIDRC/MIDRC_Diversity_Calculator
-
This algorithm uses multi-dimensional stratified sampling where several variables of interest (such as demographics - race, gender, imaging acquisition system) can be sequentially used to divide the data into numerous strata, each representing a unique combination of variables. Within each resulting stratum, patients are assigned to a specific dataset. This algorithm was developed and is used by MIDRC for separation of data into either the open data commons or the sequestered data commons. However, as shared here by MIDRC, it can be generalized by users for other needs for stratified sampling, e.g., dividing your own dataset into a two separate sets: one for training and one for testing.
Code:
COVID-specific: https://github.com/MIDRC/Stratified_Sampling
General: https://github.com/MIDRC/Generalized_Stratified_Sampling
Publication:
N. Baughan, H. M. Whitney, K. Drukker, B. Sahiner, T. Hu, G. H. Kim, M. McNitt-Gray, K. J. Myers, M. L. Giger, “Sequestration of imaging studies in MIDRC: Stratified sampling to balance demographic characteristics of patients in a multi-institutional data commons.” Journal of Medical Imaging, Vol. 10, Issue 6, 064501 (November 2023). https://doi.org/10.1117/1.JMI.10.6.064501.
-
Task based sampling begins with the identification of cases relevant for a specific task and target population demographic characteristics (such as age range, COVID status, and imaging modality). Then, optimized quota sampling is conducted by randomly sampling cases until the maximum category margin (Baughan et al. 2022) is less than a pre-specified value. N. Baughan et al., “Task-Based Sampling of the MIDRC Sequestered Data Commons for Algorithm Performance Evaluation,” presented at Annual Meeting of the American Association of Physicists in Medicine, 2022, E257–E258).
Code:
-
A document-level classifier for COVID-19 on radiology reports to help find COVID cases, as well as create large numbers of labels for computer vision models.
Code: https://huggingface.co/StanfordAIMI/covid-radbert
Publication: https://pubmed.ncbi.nlm.nih.gov/36323915/
-
An automated de-identification pipeline for radiology reports that detects protected health information (PHI) entities and replaces them with realistic surrogates "hiding in plain sight." Our model outperformed all de-identifiers as well as human labelers when it was compared on all test sets of i2b2 2014 data. It enables accurate and automatic de-identification of radiology reports.
Code: https://huggingface.co/StanfordAIMI/stanford-deidentifier-base
Publication: https://www.ncbi.nlm.nih.gov/pubmed/36416419
-
RadBERT is a transformer that was continuously pre-trained on radiology reports from a BioBERT initialization.
-
RadGraphis a tool designed to support the development of AI models that understand and evaluate radiology reports. Combining a Python-based labeling tool with an annotated dataset, RadGraph enables researchers to extract medical terms and their relationships from unstructured text. It serves as a foundation for training and refining natural language processing (NLP) models specifically tailored to healthcare applications. RadGraph is hosted on PhysioNet, accessing the tool requires user credentials.
Explore RadGraph’s code and additional resources on its GitHub page. Pre-trained models and details about RadGraph are available on HuggingFace.
Code: https://physionet.org/content/radgraph/1.0.0/
Publications: ACL 2024 Findings: Overview of RadGraph’s advancements in radiology NLP, and EMNLP 2022 Findings: Key use cases and evaluation of RadGraph’s dataset.
-
An end-to-end pipeline for the classification of chest X-rays that may belong to COVID-19 positive patients to enable real time diagnosis of the virus in the field without having to wait 24-48 hours for the results of an RT-PCR test or the less accurate results of a rapid antigen test.
Code: https://github.com/MIDRC/COVID19_Lung_Classification_CXR_Emory-ResNet50
-
COVID19 Disease Trajectory Prediction using Xrays and EHR, this model predicts a label for each chest X-ray.
Code:
-
The model is trained with JSRT data and the corresponding lung masks. The training images are enhanced and re-sized to 256 x 256 before feeding to the network. The model is trained at The Ohio State University Wexner Medical Center, Department of Radiology, using Python, Tensorflow Keras API, and trained on an NVIDIA QuadroGV100 system with CUDA/CuDNNv9 dependencies.
Code:
https://github.com/MIDRC/COVID19_Lung_Segmentation_CXR_OSU-UNet
-
The model is trained with CT sequences and the corresponding lung masks. The training images are enhanced and re-sized to 256 x 256 before feeding to the network. The model is trained at The Ohio State University Wexner Medical Center, Department of Radiology, using Python, Tensorflow Keras API, and trained on an NVIDIA QuadroGV100 system with CUDA/CuDNNv9 dependencies.
Code: https://github.com/MIDRC/COVID19_Lung_Segmentation_CT_OSU-UNet
-
ViLMedic is a modular framework for vision and language multimodal research in the medical field.
This library contains reference implementations of state-of-the-art vision and language architectures, referred as “blocks” and full solutions for multimodal medical tasks using one or several blocks.
Code: https://vilmedic.app/, https://github.com/jbdel/vilmedic
-
RoentGen is a generative vision-language model to create chest x-rays based on radiological text inputs.
-
A classification model for COVID-19 detection on Chest X-Rays.
Code: https://github.com/MIDRC/COVID19_Lung_Classification_CXR_DenseNet
-
The American College of Radiology developed a chest x-ray COVID-19 classification algorithm by training on the labeled CXR MIDRC data.
Code: https://github.com/MIDRC/COVID19_Lung_Classification_CXR_ACR
-
Notebooks and materials for cohort building for MIDRC Grand Challenges. The COVIDx challenge concerned the classification of portable chest radiographs for COVID-19. The mRALE Mastermind Challenge involved AI to predict COVID severity on portable chest radiographs.
Materials for COVIDx: https://github.com/MIDRC/COVID19_Challenges/tree/main/Challenge_2022_COVIDx
Materials for mRALE Mastermind: https://github.com/MIDRC/COVID19_Challenges/tree/main/Challenge_2023_mRALE%20Mastermind
-
MIDRC AI Interface for Covid (MAIIC) provides an interface for easy prototyping and testing of AI algorithms for AI researchers and physicians.
-
MIDRC collaborators at Argonne National Laboratory developed the Advanced Privacy Preserving Federated Learning (APPFL) framework for federated learning scenarios in which data privacy can be maintained across communication through differential privacy.
Code:
Coming soon!
Documentation:
-
The MIDRC-LOINC mapping table serves as a tool for standardizing DICOM metadata, particularly for secondary research endeavors such as AI studies. By translating DICOM image terms into LOINC codes and Long Common Names, this resource streamlines cohort selection based on essential attributes like body region and contrast presence. Its regular updates, managed by the MIDRC Data Quality and Harmonization subcommittee, ensures ongoing relevance and utility for the broader research community.
Code:
-
Jupyter or R notebooks that demonstrate how to build cohorts via queries and access associated metadata and files in MIDRC using Python or R code.
Code: https://github.com/MIDRC/tutorial_notebooks
Where to find in the data portal: https://data.midrc.org/resource-browser
-
The RSNA DICOM Anonymizer is a free open-source tool for curating, de-identifying and transferring imaging datasets. The Anonymizer program has versions for major OS platforms (MacOS, Windows, Linux Ubuntu) designed to perform "on-prem" de-identification of imaging datasets for use in research. Written in Python, using widely adopted libraries for processing medical images, Anonymizer is designed to be extended for specific project needs.
-
The COVIDx challenge task was the classification of portable chest radiographs for COVID-19.
First place: Ran Zhang, Dalton Griner, Guang-Hong Chen
Second place: Mathieu Goulet
Third place: Finn Behrendt
-
1st place: Ian Pan (Brigham and Women’s Hospital)
2nd place: Ran Zhang (University of Wisconsin-Madison)
code: currently not available due to potential regulatory approval
3rd place: Finn Behrendt (University of Technology Hamburg)
4th place: Team: Christian Mattjie, Luis Vinicius de Moura, Rafaela Cappelari Ravazio, Otavio Parraga, Luca Silveira Kupssinskü, Adilson Medronha, and Rodrigo Coelho Barros (Pontificia Universidade Católica do Rio Grande do Sul)
5th place: Yijie Yuan (Johns Hopkins Medical)
6th place: Team: Cohen Archbold, Imran Abdullah-Al-Zubaer, Atik Ahamed (University of Kentucky)
7th place: Mathieu Goulet (Centre régional intégré de cancérologie)
8th place: Team: Yifan Wu, Hayden Gunraj, Chengzong Zhao, Yuhao Chen, Alexander Wong, Pengcheng Xi (University of Waterloo)
9th place: Team: Stanley Liang, Sameer Antani, Zhiyun Xue, Sivaramakrishnan Rajaraman, Feng Yang (NIH National Library of Medicine, Computational Health Research Branch)
Questions? Check out our answers to frequently asked questions!
How to acknowledge 1) MIDRC funded research and 2) use of data downloaded from the MIDRC Data Commons